Isaac Text Labelling & Annotation Project
The advent of Artificial Intelligence (AI) and Machine Learning (ML) has brought about a significant shift in industries across the globe. One of the key elements driving these technologies is data, specifically annotated data. This case study delves into a project executed by MoniSa Enterprise, focusing on text labeling and annotation, a crucial component of data preparation for AI and ML models.
Project Overview
The Isaac Text Labelling & Annotation Project commenced on December 22, 2022, and concluded successfully on January 31, 2023. The project focused on the annotation of various types of data, with text being the core. The key objectives revolved around accurate data labeling catering to specific annotation requirements such as object detection, sentiment analysis, and named entity recognition, all while handling a large volume of data within tight timelines.
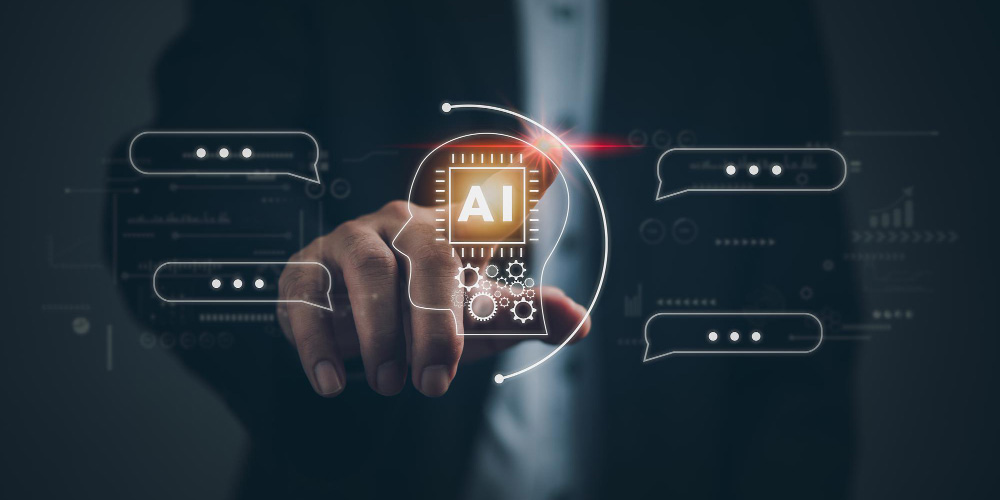
Service Category
The service extended by MoniSa Enterprise falls under the category of Text Labelling & Annotation. This process involves adding metadata to identify, categorize, and understand various objects or elements in a text.
Languages Involved
The entire project was conducted in English, which is considered a universal language and is widely used in AI and ML models.
Project Scope and Objectives
The project aimed at annotating and labeling a diverse range of data types, specifically text. The primary objectives were as follows:
- Accurate data labeling to fulfill specific annotation requirements.
- Efficiently managing a large volume of data.
- Meeting project deadlines without compromising quality.
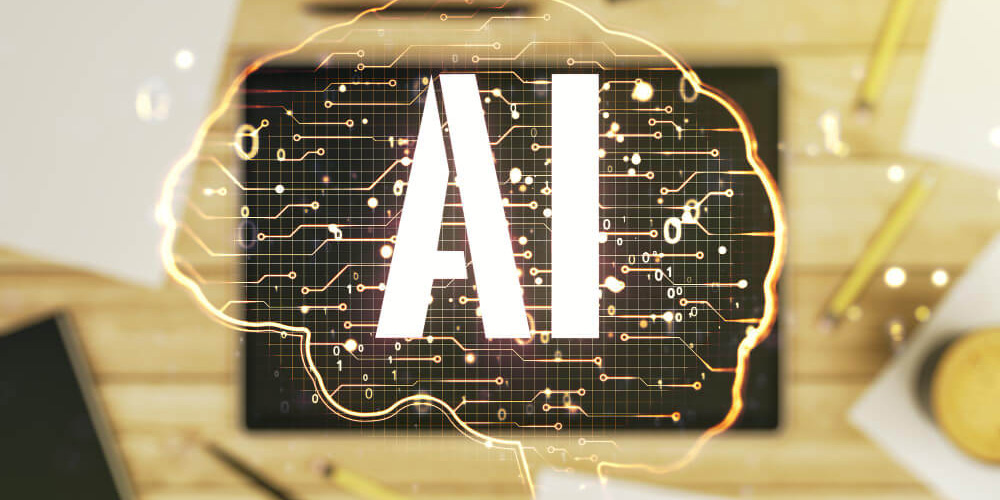
Technologies Used
The project utilized an offline client annotation tool to carry out the task. The tool facilitated efficient and accurate labeling and annotation of the text data.
Challenges and Solutions
The project utilized an offline client annotation tool to carry out the task. The tool facilitated efficient and accurate labeling and annotation of the text data.
- Ensuring consistency among annotators.
- Scaling annotation efforts to cope with increasing data volume.
- Processing unstructured or diverse data types.
- Handling ambiguous or outlier data instances.
To overcome these challenges, MoniSa Enterprise implemented several strategies:
- Training sessions: These were conducted to ensure annotators were well-versed with the guidelines.
- Calibration sessions: These sessions aimed to align the interpretations of annotators.
- Quality control measures: Rigorous measures were applied to ensure the accuracy and consistency of the annotations.
Work Volume
The project involved an extensive volume of work. Nine hundred sixty-seven hours were completed, with the time requirements varying based on annotation types.
Conclusion
MoniSa Enterprise successfully executed the Isaac Text Labelling & Annotation Project through expert annotators, robust tools, and effective strategies. The project underscores the importance of quality data annotation in AI and ML and how effective project execution can overcome challenges inherent in the annotation process.
Interested in enhancing your data annotation processes or setting up a robust system for your AI projects? Contact MoniSa Enterprise to discover how our tailored solutions can elevate your data handling capabilities.